UX research analysis
UX research analysis is the key to unlocking your research efforts. It’s the process of systematically examining the data you’ve collected during your research study, whether through user interviews, surveys, usability tests, or other methods. By doing so, you extract valuable insights that guide your design and product decisions and enhance the user experience of your product or service. In chapter, you’ll gain a deep understanding of how to conduct UX research analysis effectively. Whether you’re new to UX research or a seasoned pro, this chapter will equip you with the skills and knowledge needed to extract meaningful insights from your data.
UX research guide
Understanding UX research analysis
UX research analysis involves organizing, classifying, and interpreting the data you collect during your research study – like user interviews, surveys, or usability tests – and making sense of it so you can make informed and actionable recommendations.
When you conduct a UX research analysis, you’re essentially asking:
What can the data reveal about user needs?
How are users interacting with our product?
What challenges are users facing?
How can this information shape our design decisions?
A UX research analysis tackles both qualitative and quantitative data. But whether you’re dealing with numbers or narratives, the aim is the same: to uncover meaningful patterns and themes that shed light on user behavior and product usability. These insights can then guide product teams toward addressing user needs and pain points.
What’s the difference between UX research analysis and UX research synthesis?
When it comes to navigating UX research, terms like analysis and synthesis often get thrown around. But do they really mean the same thing? Before we get into the how-to’s, it’s worth understanding the key differences between these terms.
At its core, analysis involves sorting and categorizing data. You’re dissecting raw information and organizing it into manageable pieces. In UX research, this usually involves working with qualitative data, such as interview transcripts.
Synthesis takes an interpretive route. It’s about looking beyond the data and seeking patterns, connections, and meaningful relationships. In UX research, this involves spotting themes that connect user experiences, crafting coherent explanations, and building insights. This is the phase where you form groups of notes on an affinity map, merge various themes from multiple interviews, and make actionable recommendations.
Analysis and synthesis can happen at different stages, but they often happen at the same time. Through analysis you’ll be able to identify findings, and through synthesis you can turn those findings into insights.
When should you conduct UX research analysis?
So, when should you begin analyzing all that valuable data you’re gathering? While the obvious answer might be after your study ends, it’s good practice to start analyzing a few steps earlier.
Before you even begin your research, you should have clearly defined objectives and research questions. Incorporating this step into your research plan lets you bake analysis into your study from the outset. With a clear vision, you’ll know exactly what to look for when conducting your analysis, which will prevent you from getting lost in a sea of data.
As you start gathering data, conduct on-the-go analysis. This practice helps you spot any potential issues early on. For example, say you’ve conducted a couple of user interviews – by doing some early analysis of your interview transcripts, you might notice that some of your questions are steering the research away from meeting your objectives. Because you’ve caught this early, you’ll be able to fix any issues before conducting further interviews.
Starting your analysis early can also save you time at the end of the project. But it’s worth noting that most analysis will happen at the end of your study. By this point, you should have your raw data, tagged notes, and initial analysis ready – now, it’s time to dive deep.
We’ll get into the specifics of how to conduct this analysis shortly, but first, let’s explore the various types of analysis you can do.
Quantitative vs qualitative UX research analysis
As we explored in the UX research methods, there are two types of user research: quantitative and qualitative. Each approach serves its purpose and requires specific techniques to make sense of the data.
Quantitative research deals with objective, measurable data – think completion rates, task durations, and error rates. It’s all about finding concrete answers to questions like “How many users successfully completed a specific action” or “How long did it take them to navigate through a particular feature?”. This type of analysis unveils the “what” and “how” of user behavior, shedding light on user actions and interactions within a product or service.
e uncovers subjective experiences using words. This method captures the depth of user interactions – their feelings, opinions, and thoughts. When analyzing qualitative data, the goal is to identify recurring themes and patterns. This kind of analysis uncovers insights about what resonates with users, how they emotionally connect with an experience, and where they encounter challenges. Bearing in mind the depth of insights means analyzing qualitative data often takes more time compared to quantitative analysis.
Depending on your research objectives and questions, you might opt for one method or find value in combining the two. While they lead to different insights, the ultimate goal remains the same – to understand your users and how to optimize their experience.
Tips for conducting quantitative research analysis
In the world of UX research, quantitative analysis deciphers patterns to unveil the “how” and “why” behind user interactions with your product.
Below are some top tips on how to analyze quantitative data.
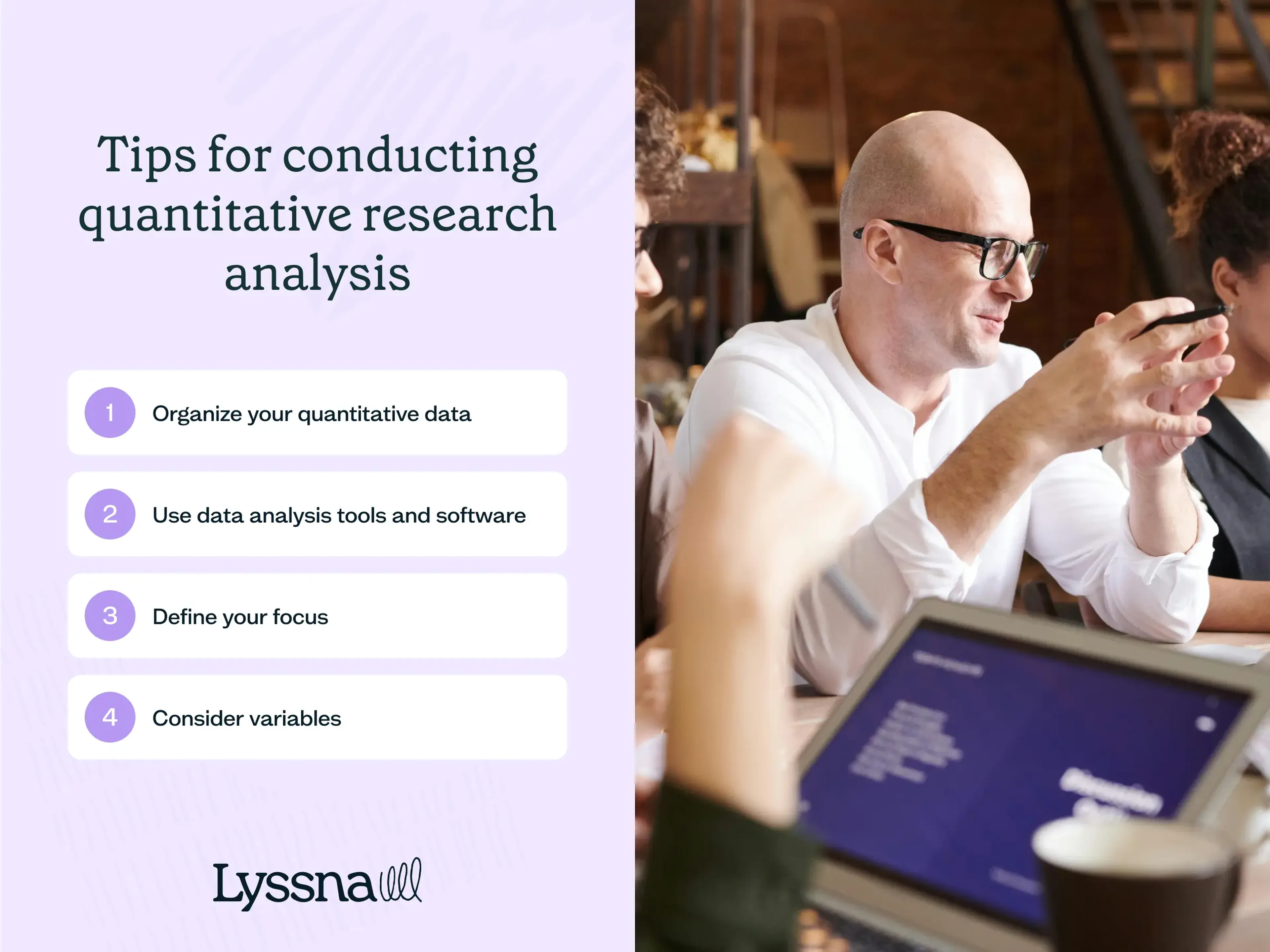
Organize your quantitative data
When you conduct a quantitative study, you’ll find yourself with lots of data. You might have the data neatly arranged in a spreadsheet, with each column representing a question and each row containing a participant’s responses, or you might need to manually compile data from various sources. Either way, organizing your datasets in a manageable way will help bring structure to your analysis.
Use data analysis tools and software
Once your data is neatly organized, it’s time to undertake statistical analysis. Tools like R, Python, SPSS, and Excel can help you crunch the numbers and unearth valuable insights.
Most user research tools will have some analysis functionality built in. In Lyssna, there are various reporting and analysis features available to make this task easy to manage.
Define your focus
Before you start drawing any insights, revisit your study’s core objectives. What questions are you seeking to answer? For example, you might want to determine how long it takes for a user to complete a task or gauge their satisfaction at different stages of a checkout process. Concentrate your analysis on these key questions.
Consider variables
Common variables explored in quantitative analysis include success rates, task completion times, and error rates. You might also assess the overall user experience through survey responses or behavioral data. Demographic and geographic data can also be folded into the analysis, offering insights into user patterns among specific groups.
Tips for conducting qualitative research analysis
Qualitative research analysis involves carefully reviewing non-numerical data collected from sources like user interviews, focus groups, or diary studies. Here’s how to best approach it.
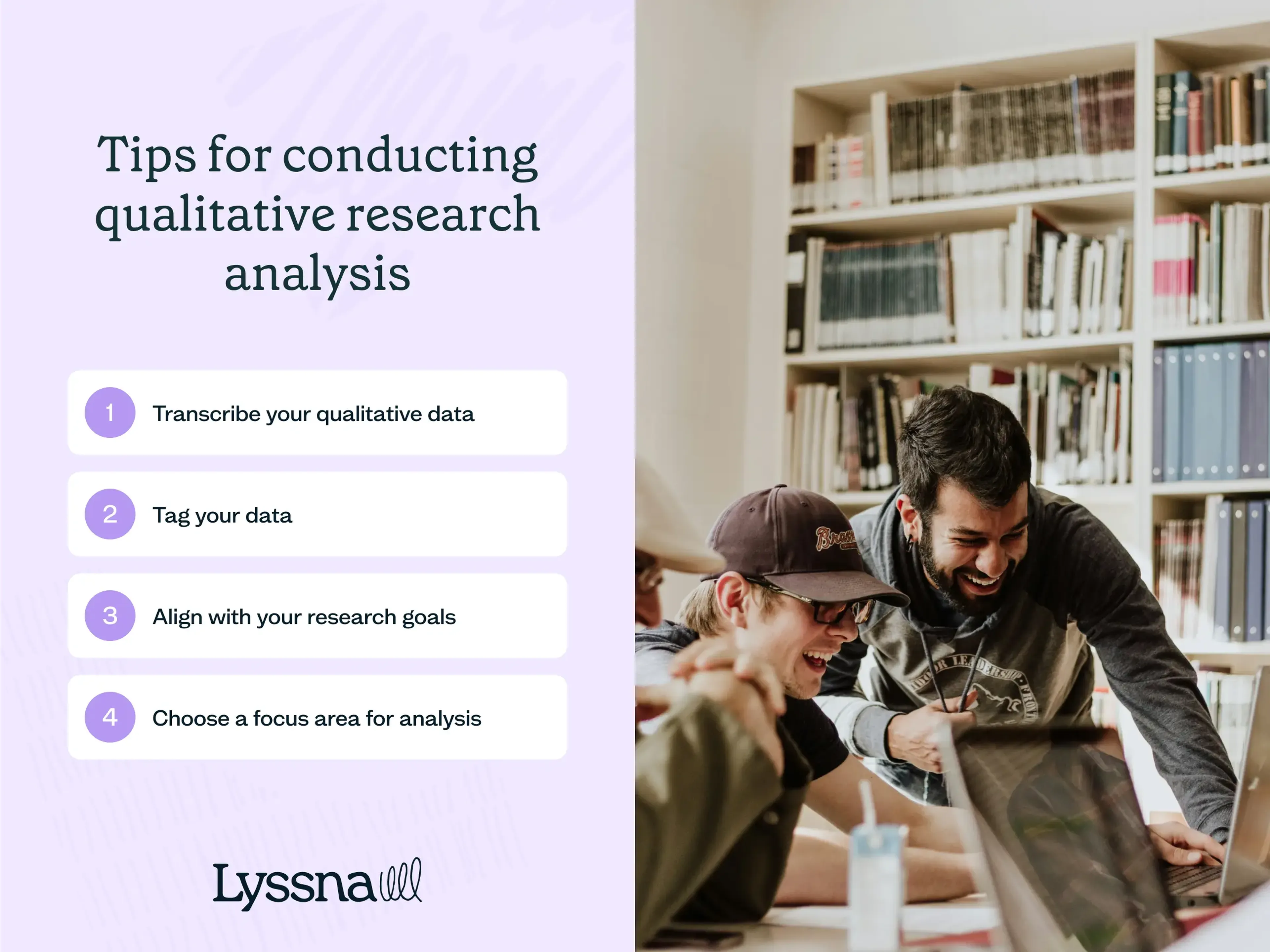
Transcribe and tag your qualitative data
If your research involves spoken or written responses from participants, such as interviews, you’ll need to transcribe this data. Transcription tools like Rev and Otter can streamline this process. In Lyssna, automated transcripts for user interviews are built in.
You’ll also need to consider how you’ll tag your data. Some UX research tools, like Dovetail and UserTesting, include transcription and tagging features, which can also help streamline this process. Other popular tagging tools include Miro and Airtable.
Align with your research goals
Before you dive into your analysis, revisit your research objectives to make sure your analysis aligns with them. For example, if your goal is to understand user preferences regarding a product’s user interface, focus your analysis on responses relevant to this goal.
Focus areas for qualitative analysis
To make your qualitative analysis more effective, consider the following focus areas as you explore the data:
Patterns and themes: Look for significant patterns and common themes that emerge from users’ responses. These recurring elements often hold valuable insights.
Surprising findings: Take note of any unexpected findings. Investigate these surprises to uncover their potential implications and significance.
Emotional responses: Pay attention to the contexts in which users express significant emotional responses to questions. Emotions can reveal critical aspects of the user experience.
Feature importance: Determine which product features hold the highest importance for users. Insights into feature prioritization can guide future design decisions.
Likes and dislikes: Explore what users like most and least about the product, and delve into the reasons behind these preferences. This can highlight areas for improvement.
User values: Examine the core values that hold significance for users in the context of your research. This can inform product alignment with user values.
User groups: Explore how responses differ across groups – this can provide insights into diverse user needs and preferences.
User stories: Seek out intriguing user stories – they can provide an interesting narrative that illustrates user experiences and challenges.
Overall product perception: Look for responses to how users perceive the product as a whole and where it fits into their daily lives. This can help you understand the overall user experience.
Unmet use cases: Look for any gaps that users highlight – identifying these gaps can drive enhancements in the user experience.
How to choose a suitable quantitative analysis approach
In quantitative research analysis, two popular analysis methods are content analysis and affinity mapping. These techniques help you extract meaningful insights from qualitative data.
Content analysis
Content analysis can help you uncover valuable patterns in your data and label them for deeper understanding. In UX research, content analysis is particularly useful when dealing with lots of text, like interview transcripts.
Here’s how to conduct a content analysis:
Define your codes: The first step is to decide on the codes you’ll use. You can either define codes based on topics you expect to find or let them emerge naturally as you delve into the data. For example, if you’re analyzing customer feedback, codes like “pricing,” “usability,” and “customer support” might come up.
Assign codes along the way: As you sort through the data, apply these codes. You can do this manually or use a qualitative analysis program (like the ones mentioned above) to streamline the process. For example, if you’re reviewing interview transcripts and a participant shared their frustrations with app navigation, you could apply codes like “usability” and “navigation.”
Organize codes into themes: To make sense of the coded data, group related codes under broader categories. For example, codes like “user interface” and “menu layout” might fall under the theme of “app navigation.”
If multiple team members are involved in coding transcripts, make sure everyone is on the same page. You can test this by having each team member code a small section of data and compare the results. You can then chat through any discrepancies until you reach a consensus on how to apply the codes.
Affinity mapping
Affinity mapping is a method used to transform a large volume of raw data into structured and actionable insights. This technique is especially useful when analyzing user interviews, surveys, or open-ended responses.
Here’s a brief overview of how to create an affinity map.
Collect and segment your data: Gather all the qualitative data you’ve collected. This could be notes, quotes, or excerpts.
Hold an affinity mapping session: Create physical or digital cards for each data point. During the session, have participants organize the cards into groups (you could use sticky notes or collaboration tools like Miro, InVision, or Figma).
Identify patterns and insights: As you arrange the cards, you’ll start to notice connections between different data points. Some cards may cluster together, indicating strong themes or recurring insights. This step helps you identify patterns and relationships that might not have been apparent in the raw data.
Synthesize insights and make recommendations: Once your affinity map is complete, step back and analyze the bigger picture. Synthesize the insights you’ve uncovered and use them to derive actionable recommendations. These recommendations are grounded in the patterns you’ve discovered, making them more robust and relevant to your research objectives.
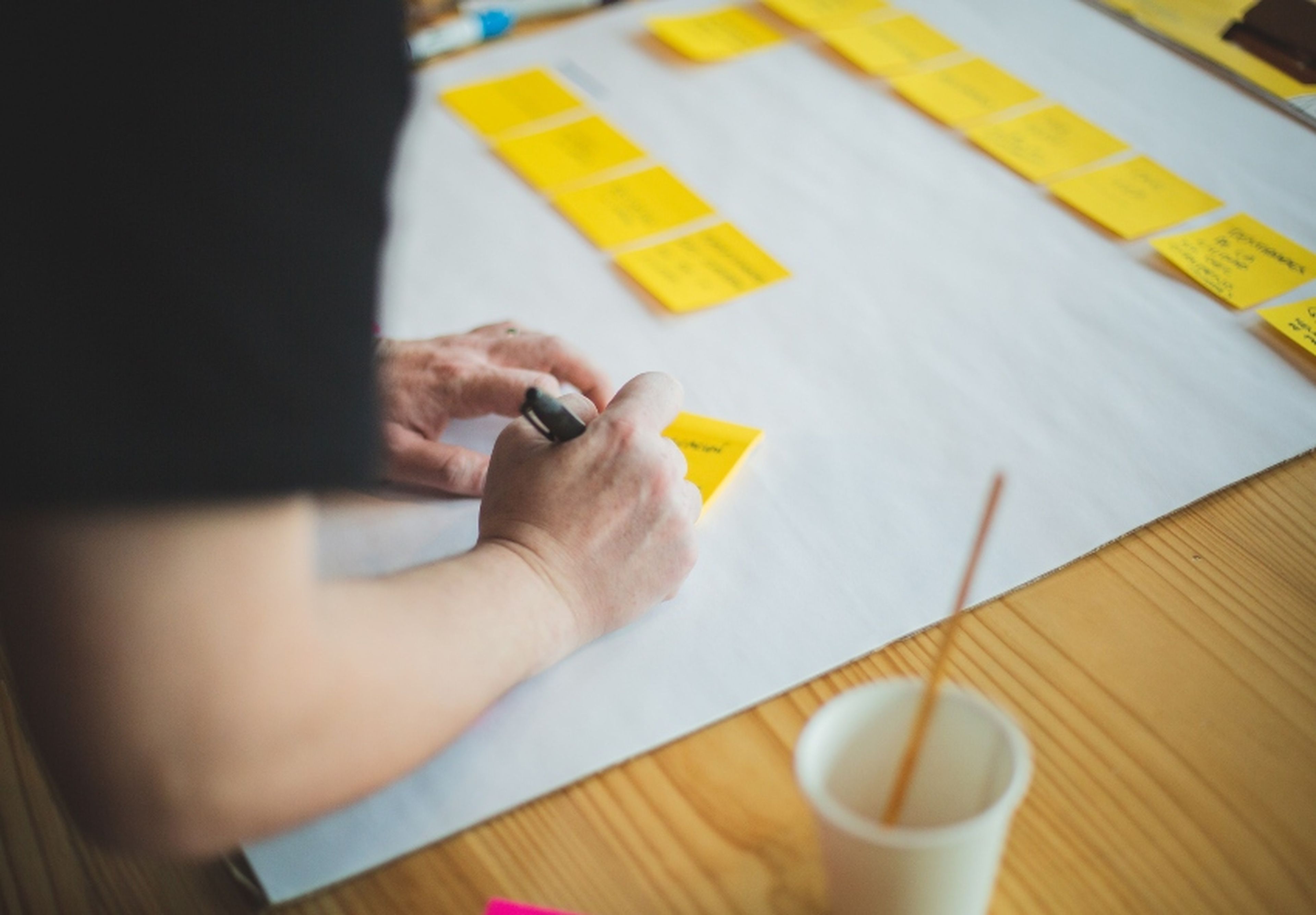
How to analyze UX research data: Step-by-step
Now that we’ve covered the different approaches you can take to analyze your UX research data, it’s time to dive into the analysis process itself. In this section, we’ll walk you through each step.
By following these steps, you’ll effectively transform raw data into actionable insights. This process not only enhances your understanding of user behavior but also equips you to provide valuable recommendations that contribute to creating more user-centered products and services.
1. Collect and organize your UX research data
We covered this in the quantitative and qualitative analysis tips above, but begin by gathering data from the various research methods you’ve used in your study, like interviews, surveys, or usability tests.
Keep things organized and structured using spreadsheets, note-taking tools, or specialized research platforms. Well-organized data is important for efficient analysis.
2. Revisit your research objectives
Before diving into analysis, revisit your initial research objectives. These objectives will help you stay on course and extract insights that directly address the aims of your study.
For example, say you’re conducting research for a collaboration tool aimed at improving remote team productivity. Your initial research objectives might include “Discover the main pain points users experience when working remotely” and “Determine which features are most valuable to remote teams.” Reminding yourself of these objectives helps you focus on aspects relevant to your research goals.
3. Uncover findings
Now, it’s time to unearth some hidden gems. This involves coding and tagging your data to categorize different types of information. Look for recurring themes, patterns, and trends that span across different data points. This might involve identifying common pain points, user preferences, or behavioral trends.
Whether you’re dealing with quantitative or qualitative data, you’re on the hunt for patterns and trends. Each finding should be prioritized based on severity and importance, always aligned with your research objectives.
Top tip: Coding data is a useful technique, especially for structuring lengthy text like interview transcripts. It involves tagging relevant insights and observations with concise phrases. For example, if a user mentions difficulties with the checkout process, you could use “Checkout issues” as a code. You can use these codes to categorize and structure your data, making it easier to identify patterns and draw meaningful insights during analysis.
4. Synthesize your findings
With your patterns and trends identified, it’s time to weave them into a coherent narrative. Group related findings together to create insights into user behavior, needs, and motivations. As we explored at the start of this chapter, synthesis is all about crafting a comprehensive story that showcases the bigger picture and the connections between various insights.
What’s the difference between findings and insights?
Findings and insights are often used interchangeably, so let’s take a quick moment to understand the difference between these terms.
A finding is a fact or statement that describes what’s happening, while an insight delves into human behavior or user motivation, guiding potential solutions.
For example, a finding might be “Users abandon the checkout process due to excessive form fields.” An insight from this finding might be, “Simplify the checkout process to enhance user satisfaction.”
Top tip: Consider various approaches for synthesizing your data, such as storytelling, empathy maps, or brainstorming sessions. Your aim is to transform data into actionable insights.
5. Make recommendations
Your insights hold the power to drive change and improvement. Translate these synthesized insights into actionable recommendations. Align each recommendation with your initial research objectives and outline their potential impact on user experience and business goals. Provide a hierarchy of recommendations based on importance and feasibility.
Use user statements, “how might we” questions, and video clips of users to translate complex insights into plain language. If insights are feasible, desirable, and viable, they can initiate new design and marketing initiatives. You might even be asked to weigh in on briefs that will kick off these projects and even help during development to make sure they align with user needs.
Now, your insights are ready to be shared with your stakeholders and your team! User research comes full circle when insights guide decision-making. Share your findings according to your organization’s culture, whether through a written report, a presentation, a workshop, or a combination of these approaches. We delve into the specifics of reporting in the UX research report chapter.
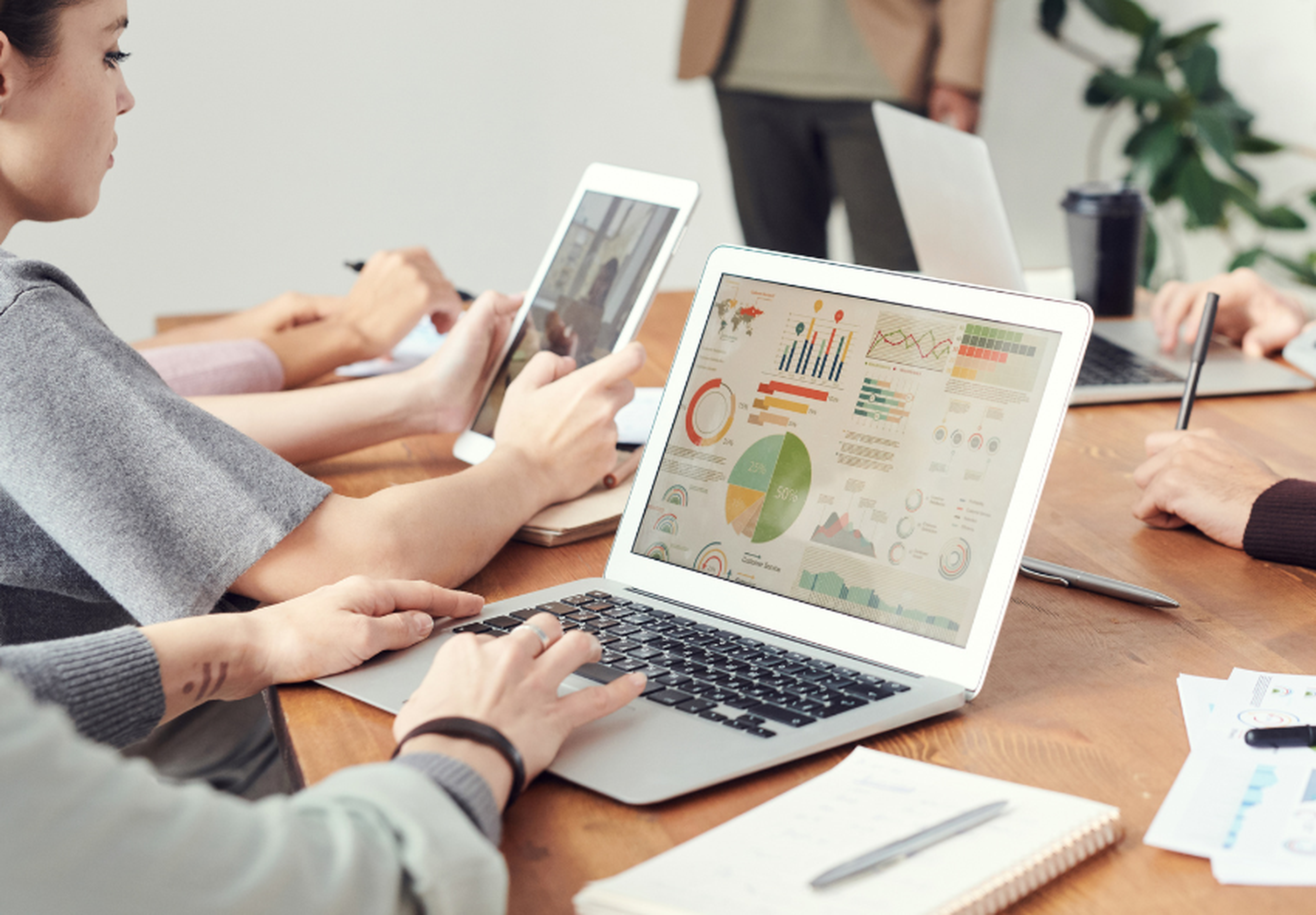
Navigating UX research analysis
In this chapter, we’ve explored the essential process of UX research analysis, a critical phase in the journey toward creating user-centered products.
Here are some key takeaways:
Analysis vs synthesis: Understanding the distinction between analysis and synthesis is crucial. While analysis involves categorizing data, synthesis uncovers patterns and relationships in that data.
When to start your analysis: If possible, start your analysis early, aligning it with your research objectives. This can save you time later down the track, as can conducting on-the-go analysis to spot potential issues.
Quantitative vs qualitative analysis: Each approach offers unique insights into user behavior and experiences. Quantitative analysis offers an objective view of user behavior, looking at the “what” and “how” of user interactions. Qualitative analysis deals with non-numerical data, delving into the “why” behind user behavior.
Equipped with these insights, you can now confidently navigate the intricate landscape of UX research analysis.