13 Dec 2024
|10 min
The role of AI in qualitative research
Learn how UX researchers are using AI tools in 2025, what the key limitations are, and why skilled researchers remain essential for effective AI implementation in qualitative research.
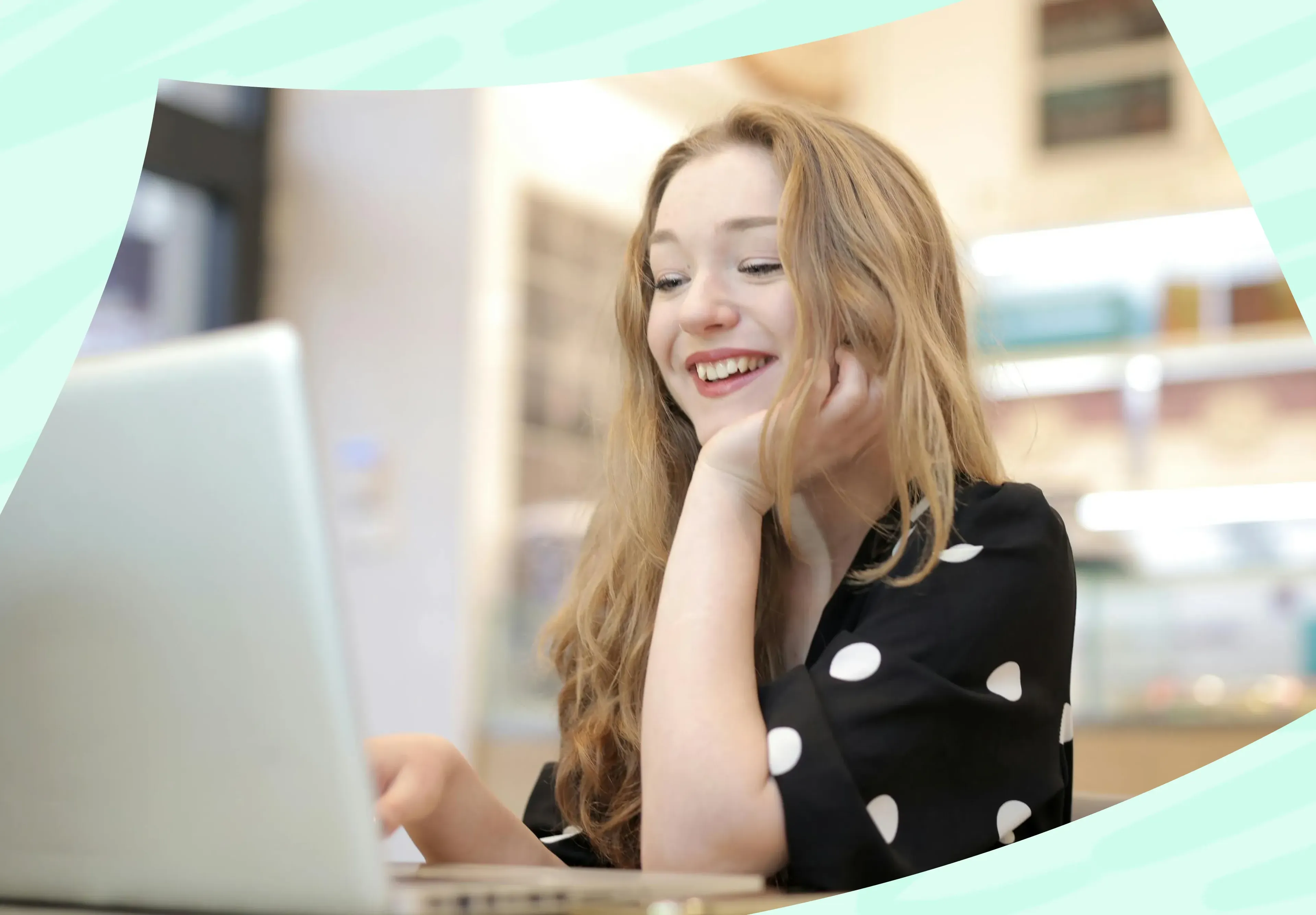
Whoever said curiosity killed the cat was dead wrong. I’m a nearly bottomless pit of curiosity and recently itched a few of those scratches.
I was particularly curious to learn how applied researchers are using AI in their work. More specifically, I wanted to understand what's working well regarding AI, what’s not, and why.
I was also dying to try AI-assisted research platforms to see how well they performed. I also knew many researchers would be interested in participating so they could “see” and “feel” the experience, too.
Fast forward a few months, and I partnered with a colleague, Dr Ari Zelmanow, on a self-funded study called "Understanding AI in Applied Research."
How researchers are using AI in 2025
Our study was based on:
My two years of AI, machine learning (ML), and natural language processing (NLP) research experience.
Using AI platforms for personal and professional needs.
The 100+ responses we received from researchers, PWDRs (people who do research), and the founders of the platforms we tested.
Taking an AI course.
My current engagement, leading generative and evaluative studies focused on enterprise AI solutions.
So, what did we find out? Not surprisingly, AI has already made a significant dent in UX research, particularly in streamlining and enhancing various aspects of the research process. The most common tasks include using AI for:
Brainstorming
Background research
Transcription
Rudimentary analysis
And the most common tools our participants mentioned included:
Bard
Butter
ChatGPT
Claude
Descript
Dovetail
Notably
Otter
Perplexity
Prolific
Reduct
Rev
Temi
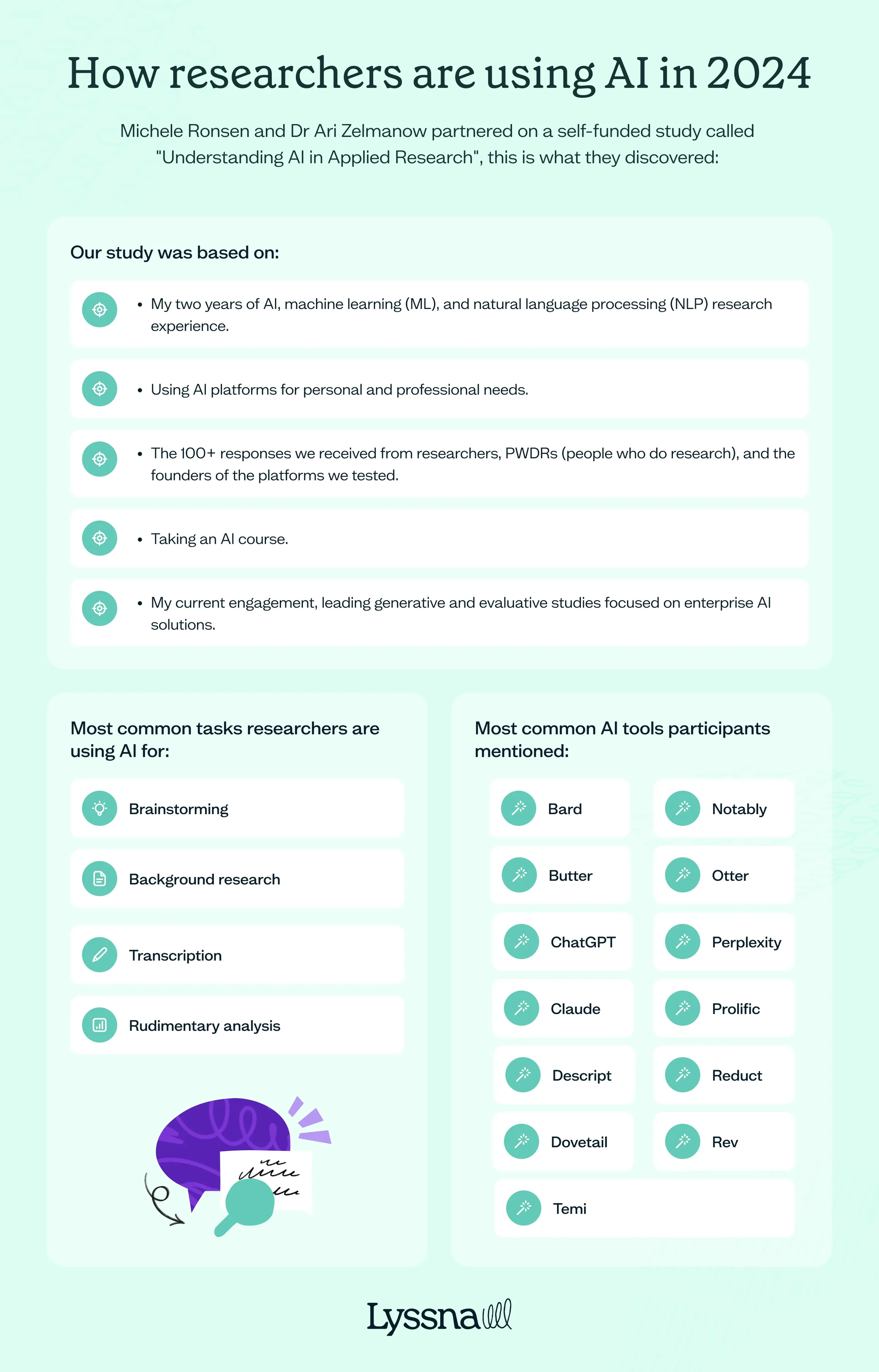
Some other AI platforms offer more promising capabilities specifically for conducting AI-assisted research, but most are still trying to achieve product-market fit. The research community we engaged with is taking advantage of these tools, albeit to widely varying degrees. That said, it's crucial to understand that while AI can assist with qualitative research, it’s far from replacing the expertise of trained researchers.
Leveraging AI effectively demands more nuanced research skills and a deeper understanding of research practices. We heard this loud and clear, over and over again. My own professional experiences also echo this sentiment.
The limitations of AI in qualitative research
Yes, AI has made impressive strides in supporting qualitative research tasks. For instance, AI can streamline brainstorming sessions by generating creative ideas, assist in decoding complex acronyms, and provide quick background information.
Additionally, it can optimize processes by producing accurate transcriptions and language translations, generate summaries with timestamps, and highlight key segments from interviews or unmoderated sessions.
However, AI's current capabilities aren’t yet sufficient to replace a trained qualitative researcher. From what we experienced, and heard from our participants, they aren’t even a close second due to a few reasons:
Generative AI tools, such as ChatGPT, struggle when it comes to analyzing and synthesizing qualitative data. The two AI-assisted research tools we tested offered only a basic, first-time, untrained approach. While they’re proficient at generating text based on patterns in the training data, they can’t inherently understand or interpret data like a human.
They’re unable to consider context outside the data sets they have access to, which poses a significant challenge in utility, and a fundamental gap in regards to outputs.
As a result, the AI tools we tested aren’t yet capable of handling the complex analysis and synthesis required in most qualitative research.
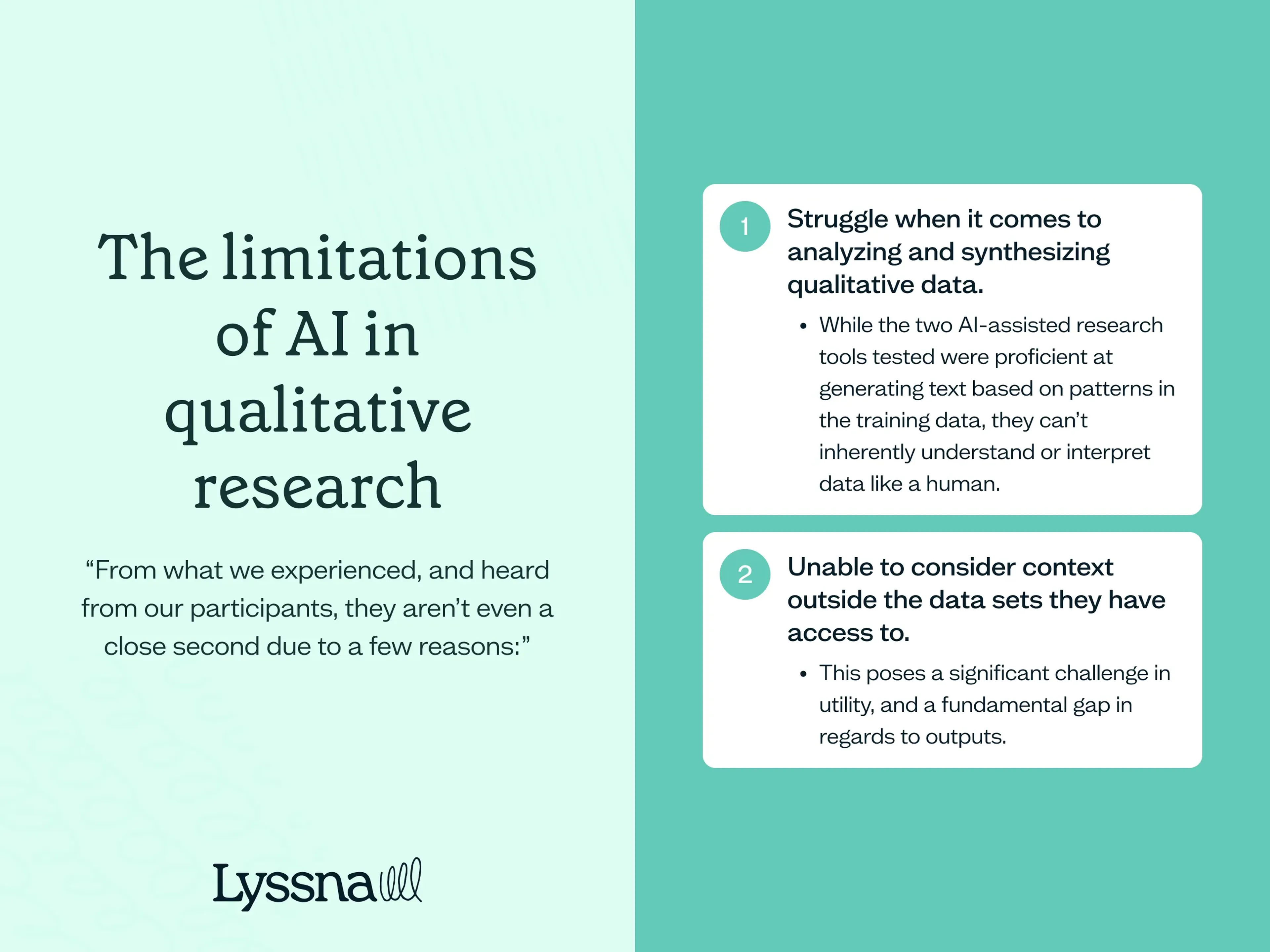
Why skilled researchers are key to using AI effectively
The effectiveness of generative AIs is intrinsically linked to the quality and breadth of the data it’s connected to or has been trained on. These tools are only as strong as the information they can access, which means their outputs are compromised if the necessary data is missing, insufficient, or biased.
This is a critical consideration if you’re looking to leverage AI in your research work, especially considering a substantial amount of our efforts are based on previous experience. Additionally, analysis, synthesis, and decision-making often require many inputs.
To optimize the use of AI platforms, you must have a deep understanding of both the research context and the AI tools themselves. Crafting effective prompts and providing relevant context are essential for obtaining high-caliber agential outputs. Without uploading comprehensive data – such as previous studies – and providing additional context, the AI’s approach will likely be incomplete and unsubstantiated.
Examples of relevant context will vary from study to study. They may relate to the surrounding culture, pertinent opportunities and barriers, internal or external factors, new and existing competition, financial models, different use cases, buyers versus users, collaboration, mental states, physical environments, and so on.
While flawed data from an AI platform may be easily apparent to a trained practitioner, they may not be as obvious to someone without years of applied research expertise.
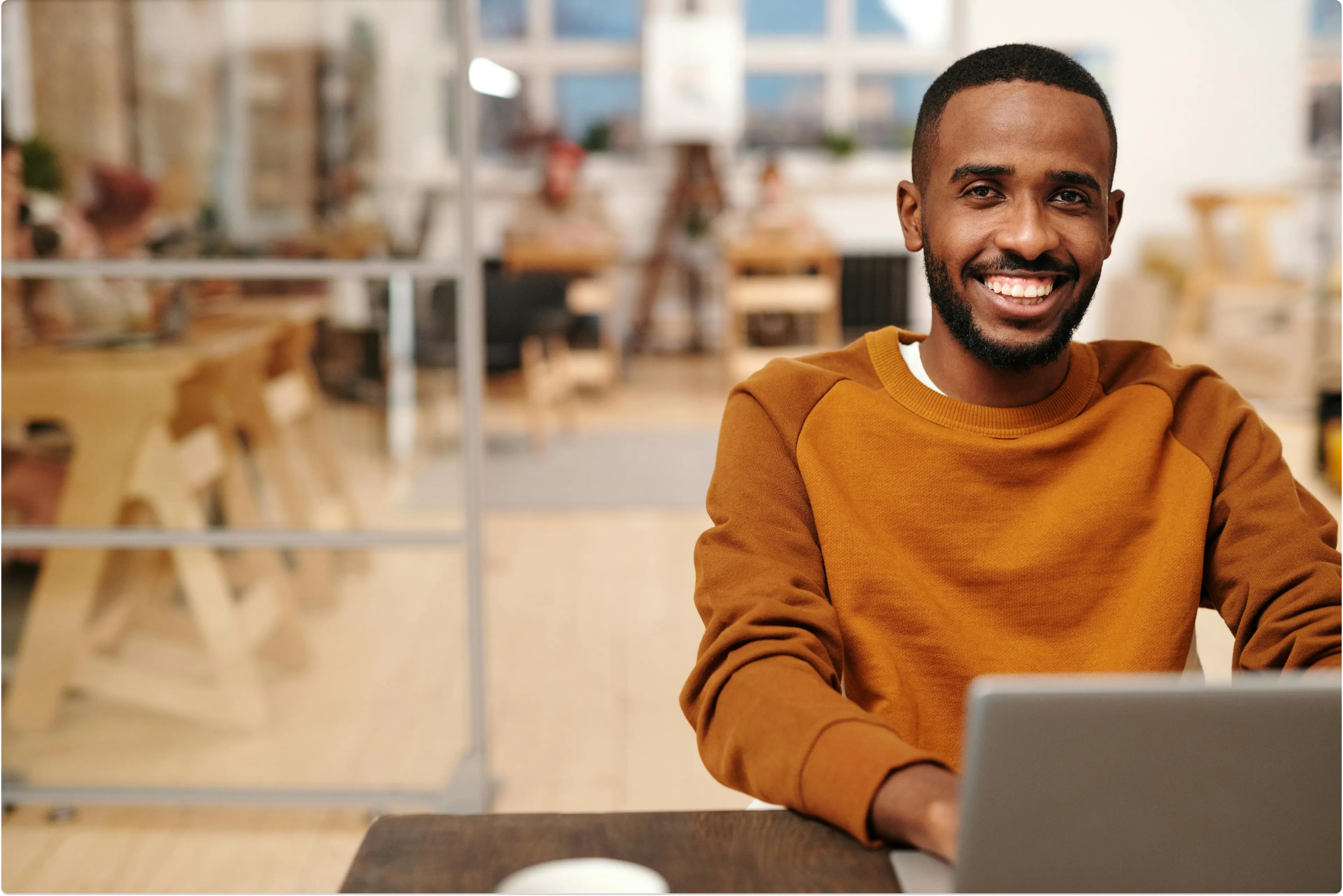
The importance of data quality and effective prompts
As I mentioned above, one of the most significant factors in maximizing AI's utility in research is the quality of the data you input and the effectiveness of the prompts you use.
AI tools need well-structured data and clear, detailed prompts to generate useful outcomes. If you don’t provide this level of detail or context, the agent's results will fall short, leading to subpar or inaccurate results.
Furthermore, AI tools aren't infallible. They can inadvertently reinforce existing biases if the training data contains them, or they might miss subtle nuances that a human researcher would catch. This underscores the necessity of a trained researcher to carefully oversee and interpret AI-generated outcomes to make surre they align with the research objectives and maintain integrity.
Here's a helpful analogy.
A trained researcher knows how to pose and sequence unbiased questions to participants in a live user interview. They know how and when to use their improv skills, deviate from their guide, identify which aspects to push and pull on (or not), how and when to revert to the core questions at hand (by redirecting the participant), and troubleshoot in the moment.
They consistently gather the inputs from participants that are required to deliver their research goals, produce sound and culturally relevant results, and deliver meaningful and actionable recommendations to stakeholders.
Optimizing AI for research requires these same skills – with one key differentiator.
The trained researcher needs to learn how to do all of this with an inanimate AI platform that may know nothing about applied research (other than what it has direct access to, which might be subpar and lacks the 360-degree necessary context). This takes A LOT of work – similar to training a human!
Knowing how to pose and sequence unbiased questions in a user interview to produce reliable and relevant results is akin to knowing how to drive AI platforms.
Future directions: Enhancing AI's role in research
As AI technology advances, its potential to enhance qualitative research, and specifically low-hanging usability studies, will certainly grow.
Since conducting this study, I’ve already found more advanced AI-assisted research platforms that promise substantive sophistication and nuance. Future developments will no doubt improve AI's ability to analyze and synthesize qualitative data more effectively.
However, even with these advancements, the role of researchers will remain crucial, if not even more important. In addition to training models, they’ll be needed to assist non-trained researchers in driving AI platforms to achieve feasible, accurate, and actionable results. Researchers will need to stay informed about the latest AI tools and methodologies, and they'll continue to play a key role in guiding AI’s application and interpreting its outputs.
Individual and team-customized assistants, also known as agents, are coming down the pike quickly. The technology is available today, yet most people haven't developed their agents yet.
At this point, it doesn't seem that trained researchers have reason for concern. Researcher jobs aren’t in jeopardy – in fact, there will be a greater need for skilled researchers as AI evolves.
The juice isn’t worth the squeeze – yet
AI has the potential to significantly enhance various aspects of qualitative research by improving efficiency and providing valuable insights. However, it isn't a substitute for the expertise of trained researchers.
The effective use of AI requires a deep understanding of both the technology and the research context. While tools like ChatGPT offer promising support, they aren't capable of replacing the nuanced analysis and synthesis performed by skilled researchers today.
As AI technology continues to evolve, it will be essential for researchers to stay adept at both leveraging these tools and maintaining their critical role in the research process.
If you’re interested in exploring how AI is currently being utilized in applied research, please reach out! I’m super passionate about and open to connecting with people interested in exploring how AI is being used in applied research and what the future may hold for our industry.
Level up your research practice
Ready to streamline your qualitative research workflow? Join thousands of researchers already using Lyssna. Sign up for a free plan today – no credit card required.
This article was authored by Michele Ronsen, Founder and CEO of Curiosity Tank. Michele is a user research executive, coach and educator. She teaches design and user research to people around the world. Her corporate trainings and workshops are inspired by working with Fortune 500s and start-ups for more than twenty years. Fuel Your Curiosity is her award winning, free, user-research newsletter. In 2020, LinkedIn honored Michele with a TopVoices award in the Technology category. She is the first and only researcher to receive this award.
You may also like these articles
Try for free today
Join over 320,000+ marketers, designers, researchers, and product leaders who use Lyssna to make data-driven decisions.
No credit card required